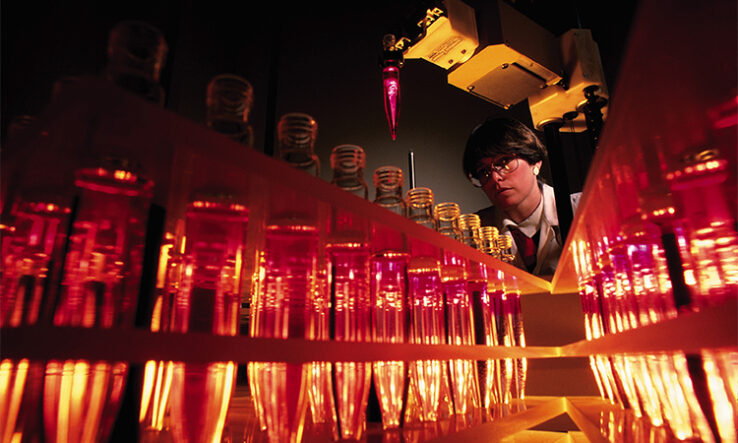
Image: William Taufic, via Getty Images
Don’t let automation threaten broad scientific training, say Kieron Flanagan, Barbara Ribeiro and Priscilla Ferri
This has been the year of generative artificial intelligence. Tools such as ChatGPT have intensified interest in, and raised expectations about, the impacts of AI in all walks of life.
Governments have increasingly stepped up efforts to regulate AI, becoming more aware than ever of the need to better understand the technology’s implications and challenges. Such is the background of the AI Safety Summit being organised by the UK government this week.
The world of research has not been immune from this frenzy of interest; even before ChatGPT became famous, there was increasing attention on the prospects for AI and robotics to transform the practices, and the productivity, of the scientific enterprise through, for instance, the adoption of machine learning or advanced liquid-handling robots.
Unfortunately, touting the adoption of AI and robotics as the solution to supposed problems with scientific productivity reduces the scientific enterprise to an input-output process. This focus on knowledge output obscures broader implications for the public value of science. Our chapter in a recent Organisation for Economic Co-operation and Development book, Artificial Intelligence in Science, looks at these broader issues for policy and society raised by the effects of AI on the scientific workforce.
The human factor
One of the prime concerns driving the emergence of science policy in the 20th century was to ensure a supply of scientists and engineers equal to the needs of the economy and society. The term ‘science base’ captures this focus on the human capacity to do research.
The science base is where new scientists and engineers, not simply new knowledge, are produced. The effort to produce Covid-19 vaccines exemplified the value of a strong science base, as it required mobilising teams, networks and human capabilities, not simply building on existing knowledge.
The public value of science depends on maintaining and developing this human, organisational and infrastructural research capacity. It underpins society’s ability to carry out problem-driven research and, through the supply of scientists and engineers, also fosters entrepreneurship and industrial innovation.
Learning by doing
People typically become researchers by doing research, learning on the job as PhDs and postdocs, gaining not only skills but also the culture and assumptions of their community. When the way in which research is done changes, so do these training and development opportunities.
Historically, technology has replaced routine labour-intensive aspects of research with automated tools. But new tools also introduce new routines and mundane tasks.
Whether what’s being automated is statistical inference or pipetting, new forms of mundane work, such as cleaning data or supervising laboratory robots, will be created. Who will perform these tasks, and how will such duties affect the broad shape of their work?
Changes to scientific work will alter the quantity and quality of the training and development opportunities in the science base. If automation means that fewer early career researchers are required, or if their roles become primarily focused on work with automated systems, scientific training and development could become more narrow.
There is also a risk that important understanding and skills may be lost. For example, some commentators have argued that the widespread misuse of statistical tests in published research stems partly from the ‘black-boxing’ of statistical analysis in standardised software packages.
Team building
We cannot understand how AI might change science without considering the human and social nature of scientific practice. New ensembles of AI and other automation technologies will reconfigure the science base, changing how scientists work together and coordinate everyday tasks. This will create both new demands for funding and unpredictable consequences for the goals of science policy.
Some commentators confidently predict that new combinations of collective and machine intelligence are set to take over the larger social processes through which a scientific community sets its research agenda, sifts competing claims and agrees which are valid. Others point out that current approaches to AI contribute little to these processes.
AI is already shaping researchers’ skill sets and routines. Funders and scientists alike need to recognise that the science base that results from the adoption of AI and new forms of automation may create new demands for public funding; at the same time, it may undermine the case for public funding by reducing the public value contained within the science base.
Kieron Flanagan, Barbara Ribeiro and Priscila Ferri are in the Manchester Institute of Innovation Research, University of Manchester
A version of this article also appeared in Research Europe