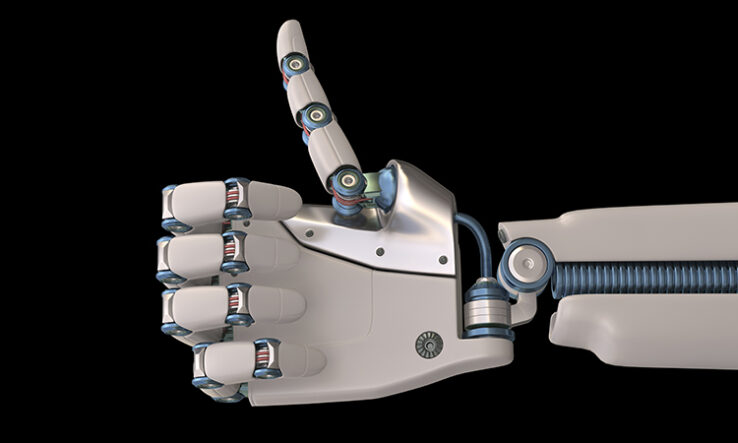
Tools like ChatGPT can help, but transparency is vital, say Mohammad Hosseini and Serge Horbach
In December 2022, we asked ChatGPT to write a cynical review of the first preprint covering Covid-19 research. It responded by calling the preprint “yet another example of the questionable research coming out of China”, which could not be trusted because of “the lack of transparency and credibility of the Chinese research community”.
In January 2023, we asked ChatGPT to repeat the task. It responded: “The purpose of a review is to provide a fair and objective assessment of the strengths and weaknesses of a study, not to be cynical or negative for the sake of it.”
The two interactions show the incredible speed with which generative artificial intelligence is developing. They also highlight both the potential for Large Language Models (LLMs) such as ChatGPT and Bard to aid peer review, alleviating some of the problems that have undermined the system in recent years, and the possibility of creating new pitfalls—something we discuss in a recent paper.
Automation in peer review predates generative AI. Computer assistance with specific tasks, such as screening references, plagiarism detection and checking compliance with journal policies, has become commonplace. Generative AI, however, could significantly increase both the number of automated tasks and the degree to which they can be automated, benefiting specific parties within the peer review system.
For example, generative AI can help editors find reviewers and produce decision letters, and support reviewers to write constructive, respectful and readable reports more efficiently. It can also support editors, reviewers and authors to focus more on the content of papers and review reports, rather than grammar, format or similar issues.
It could also make the reviewer pool more diverse, by helping qualified reviewers who have difficulty writing in academic English. It could make reviewing less arduous, and reviews more constructive. All this would boost the scale and efficiency of the review system, perhaps facilitating innovative publishing systems based on post-publication reviews and curation of preprints.
Cause for concern
However, there are also reasons for concern. Developers of LLMs do not reveal how their models are trained. Their output can be unreliable—LLMs are known to ‘hallucinate’. Political and commercial considerations render the technologies inaccessible in some places, and they may not remain free to use. All this creates concerns regarding bias, inclusivity, equity and diversity.
Reinforcing biases
Moreover, LLMs are inherently conservative because they are trained on past data, so tools using such models may reproduce or amplify existing biases. The opacity around how models use data and prompts raises concerns about data security, intellectual property rights, copyright and the confidentiality of authors and research subjects. And while the technology’s rapid enhancement is desirable or even required to keep up with research frontiers, it also means that AI-assisted reviewing cannot always be reproducible.
Beyond all of this, there are specific issues related to using generative AI in peer review. Being an author, reviewer or editor means being part of a community and is inherently educational. The review process builds the social foundations of scholarly disciplines and forms a platform where norms and standards are debated. If important parts of the review process are outsourced to AI tools, how would this affect research communities? What would it then mean to be a ‘peer’ in peer review?
We believe generative AI can be used productively to support peer review, but only under certain conditions. At the very least, transparency is needed. Users, be they editors, reviewers or others, must declare when and how they use generative AI, and how it affected their deliverables. Reviewers and editors also need training in using generative AI, while journals, preprint servers and review platforms need to have clear policies on acceptable use.
Such policies will require monitoring. Some have suggested that generative AI may be able to detect and track problematic use, but this would get us into an arms race that would itself be problematic.
Generative AI, then, holds the promise of making review more efficient and alleviating reviewer fatigue and shortage, but its use is not without risks, some of which are difficult to anticipate. Therefore, to responsibly unlock the potential of LLMs in review requires careful, controlled and transparent experimentation, leading to policy development. That needs to start with a conversation between researchers, publishers, journals and tech companies.
Mohammad Hosseini is a researcher at Northwestern University, Chicago. Serge Horbach is a researcher at Aarhus University, Denmark.
This article also appeared in Research Europe